Additionally, considering the market competition and simpler access, establishing customer engagement became one of the most efficient ways to guarantee stable revenue. The digitization of activities defined the connector - personal devices.
Everyday people (unless they prevent it) share a lot of data concerning their interests, needs, and preferences within various social networks, websites and search engines. Synchronization of data within services only simplifies access to it and the required audience for marketers.
Once the majority has its own wall of information presented voluntarily researches for defining market needs, focus groups, etc. became more accurate but more complex due to the increased amount of data, constancy, and power of its incoming flow.
Although that’s already quite enough there are still metrics concerning digital marketing and other business operations to be collected for defining an optimal strategy for a certain period and required adjustments during its running.
The number of aspects to take into account made manual statistical methods not applicable or too time-consuming for the modern pace of the workflow. That’s why business analytics turned to another field of study.
Data science in eCommerce and retail
Combining math, statistics and machine learning algorithms data science for eCommerce, retail, logistics and the whole supply chain became a game-changer. Especially when the IT market encouraged by digital transformation trends provided tools for convenient performance of the required analysis.
As a result, markets got a way to address the challenges on the core level and find custom solutions for the business. Processes automation, workflow optimization and aiming for cost-efficiency aren’t feasible without established analytics operations.
Explore what areas of the enterprise running can benefit from bringing in a data science specialist and culture in its environment.
Brand/store identification
For domains working with end-users, the positioning is vital. There’s no time to wait until focus groups will define itself or the enterprise will prove its reliability to ensure the constant flow of new customers. Data science allows determining aspects to focus on to find own niche in the market through deep analysis of the company’s model, resources, potential, location, pricing, and rival experience.
Another benefit of the scientific approach is the ability to establish data analytics from multiple sources in real-time conditions outsourcing the task to trained models. Machine learning for eCommerce is another AI subset which trends and achievements need to be applied and constantly watched for.
Customer relationship
For retail and eCommerce data science projects allow find and implement new approaches to increase brand awareness, service personalization as well as customer engagement and support. Relying not on average statistical facts but specific personal info turns annoying offerings into thoughtful suggestions lowering the potential buyer shields that now are always up due to the excessive advertising onslaught.
Any long relationship is impossible without communication. Considering the business scale to maintain it manually with the motto only custom approach is possible only within small companies. But even there it's a low-efficient way to go. Data science and AI allow automating the most significant and tedious part of building connections with new and returning customers keeping visible only efforts through human touch.
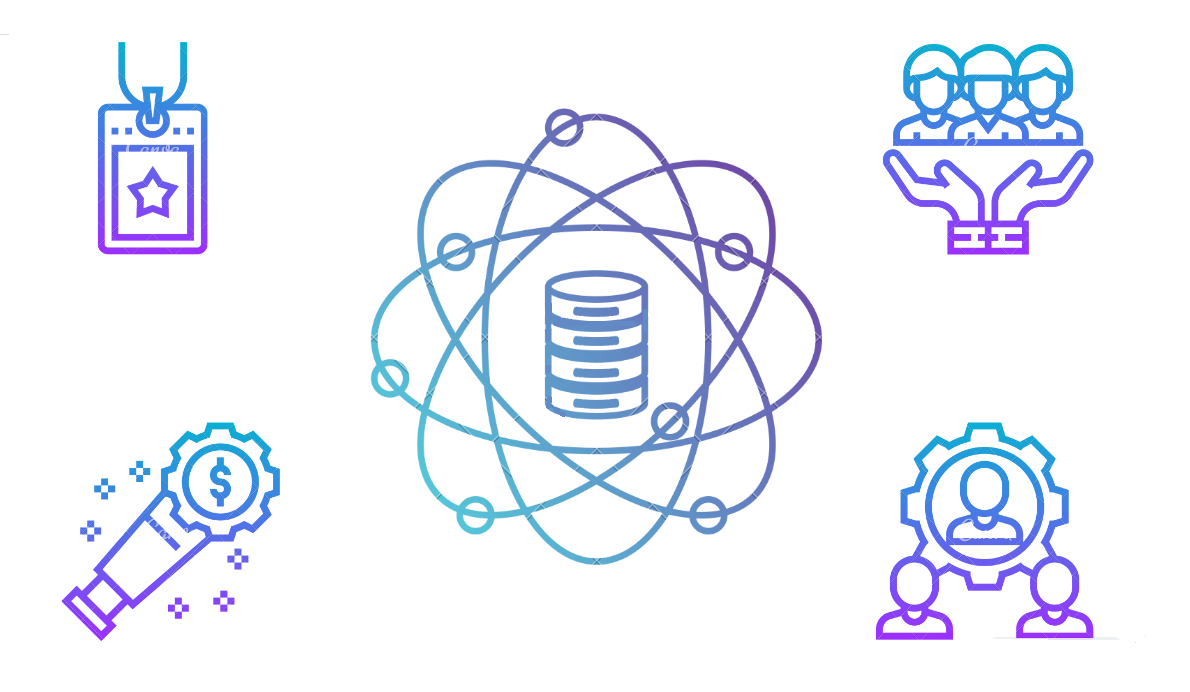
Activity forecasting
Tracking models of human behavior gives scopes like eCommerce and retail a relatively stable environment to develop in. Since domains became customer-oriented buyer activity forecasting digital or real no less significant in strategic planning than competitors moves or possible risks. The mechanism also found it’s application as an influencing remedy that has already proved its efficiency.
Predictive recommendations are a beneficial way to guide a visitor around an online marketplace offering products or services based on the prior queries or overall preferences. It allows raising the user session duration, item discoverability, engagement and ergo revenue - proven by Amazon. Based on ML algorithms engine offers are always correspond with current needs due to the regular data update.
Workflow management
Comprehensive research and big data analytics with the appropriate accuracy level make possible to detect, label and resolve problems hidden from customer attention. Arranging partnerships, storing and tracking inventory, ensuring data and items security, staff control on the matters of performance, integrity, conformity with company policy, etc. can be improved by aiming to the issue reason not display.
Nowadays popular AI-based solutions for fraud-detection, theft prevention, inventory management, enterprise resource planning (ERP) would be worthless for business workflow optimization without collecting and properly processing required data For retail and eCommerce data science models allow implementing these processes customized for the company needs.
Simply stating the collaboration of data science and eCommerce turn the "number" masks from metrics and hidden status from thoughts of store visitors making possible to elicit and comprehend their value. The knowledge makes possible to map up a real-life direction for the business and make it flexible to satisfy the needs of the constantly changing market.
Data science use cases in eCommerce and retail
Driven by data-expressed experience no less than technology accelerating field digital transformation forces to have the field of retail and ergo eCommerce data science ideas. Although it should be taken into account their implementation can be challenging and requires from the enterprise established running model, data infrastructure and, of course, noticeable financial contributions.
Nevertheless, the proven advantage of companies that haven’t been stopped by the initial difficulties works as a proclaimer of the field direction of the upcoming years: towards data science. The following data science use cases in retail both online and land-based have gained appreciation from the scope representatives allowing them to taste the fruits of the novelties of the technical progress.
eCommerce product recommendations
WHAT: Recommendation engines with either collaborative or content-based filtering that supplies online store visitors with suggestions of possibly required items.
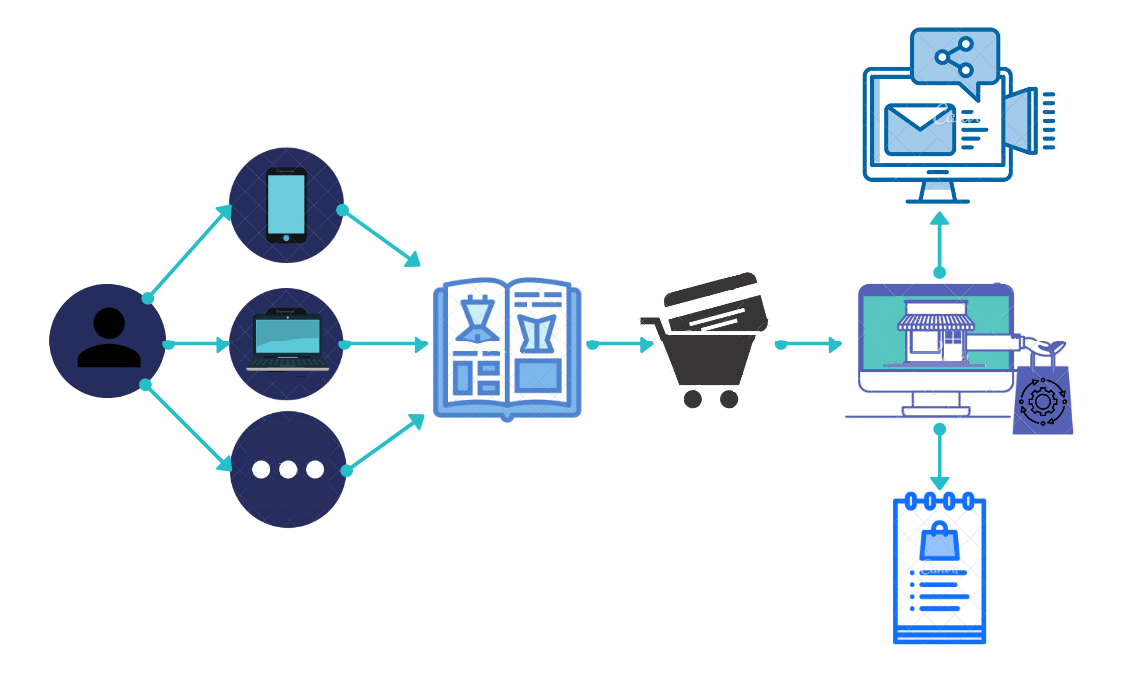
HOW: An appropriately trained machine learning model collects and analyzes data concerning previous search history or buying choices and provides similar, related or compatible products separately.
WHY: It shows a custom approach and raises the possibility of additional purchases within the same or the next shopping cart.
Customer retention solution
WHAT: Churn model that detects customers whose engagement level is lowering and soon will result in breaking the connection in favor of another store.
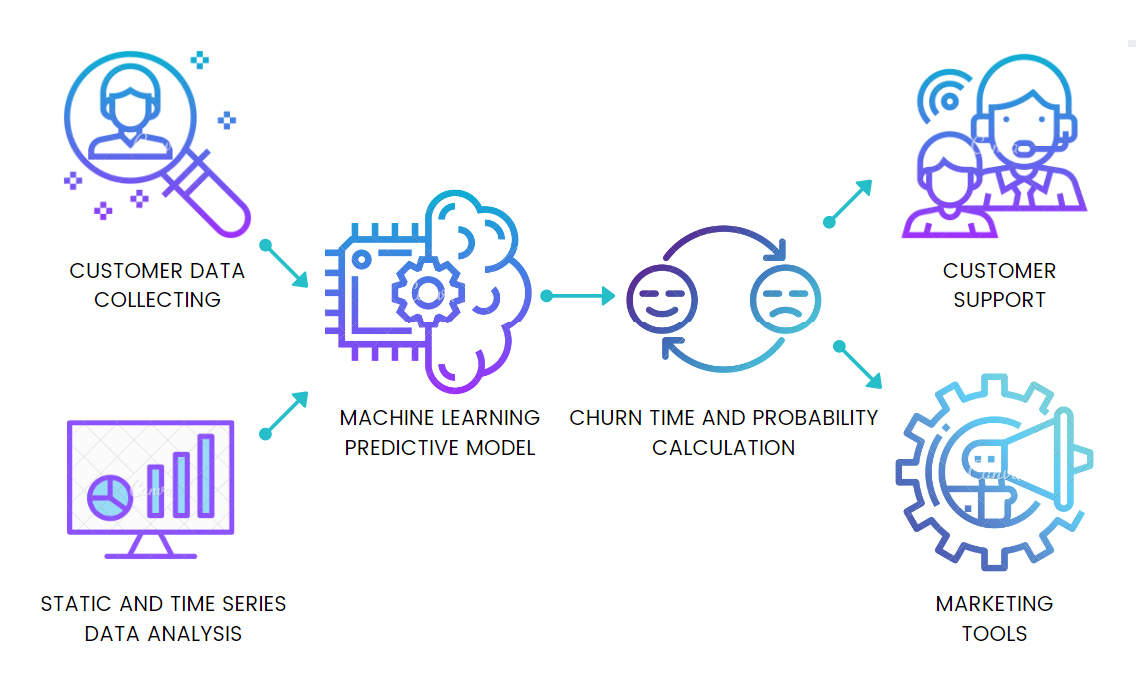
HOW: By analyzing customer activity and interaction the algorithm is able to distinguish about-to-go ones, possible reasoning and collect metrics to calculate churn metrics for further analysis.
WHY: To prevent losing existing clients using personalized marketing methods and ensure a positive tendency in their quantity and efficiency of advertising campaigns.
Lifetime value estimation
WHAT: A calculation of the possible financial contribution through purchasing to the store revenue during the customer lifecycle.

HOW: By collecting, analyzing and multiplying readings of numbers, average costs, and span - metrics that define customer lifetime value (CLV) using an ML model.
WHY: To raise the level of understanding of the focus group by identifying personal buying patterns to adjust existing and future marketing and business development strategies.
Fraud/theft prevention
WHAT: Digital solutions that allow minimizing risks of stealing personal data, goods or money or collapsing a certain process.
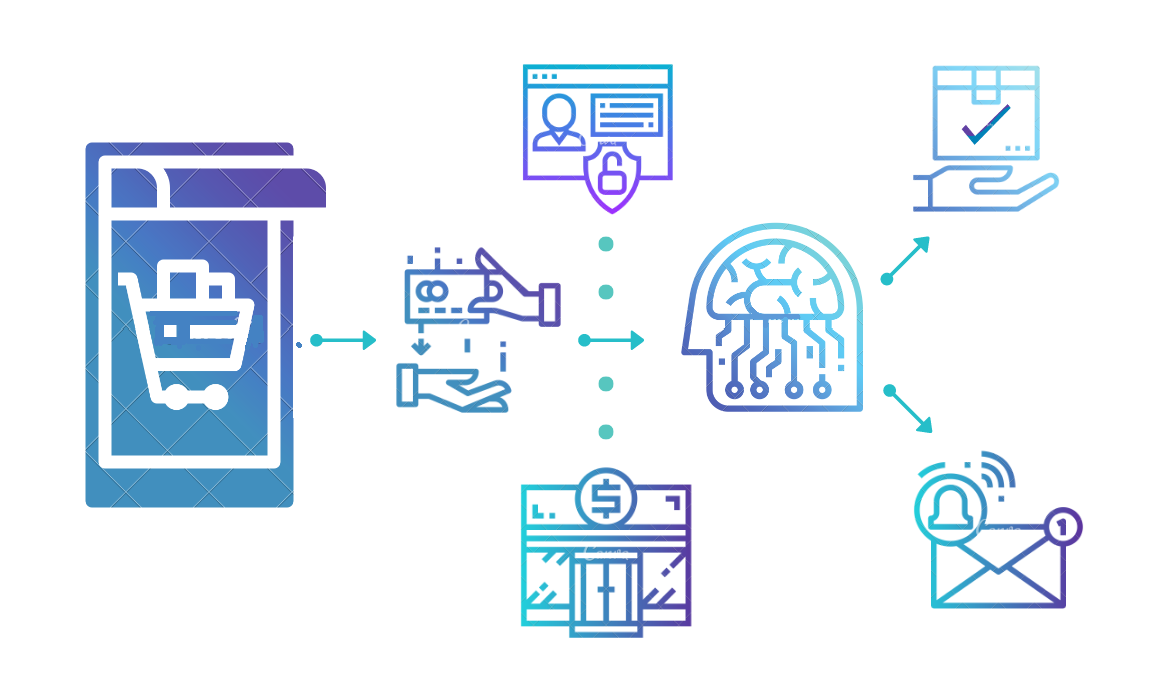
HOW: By applying data science techniques like neural networks, clustering, data mining, etc. to detect unusual buyer, employee or system behavior according to determined models and block wrong actions.
WHY: To increase facilities transactions and data security and protection, prove reliability to assure sufficient customer retention rates and lower related expenses.
Pricing/loyalty optimization
WHAT: An adjustment based on analysis of customer feedback, rival rate politics and other specifics like location, seasoning to implement a dynamic pricing model or improve customer service.
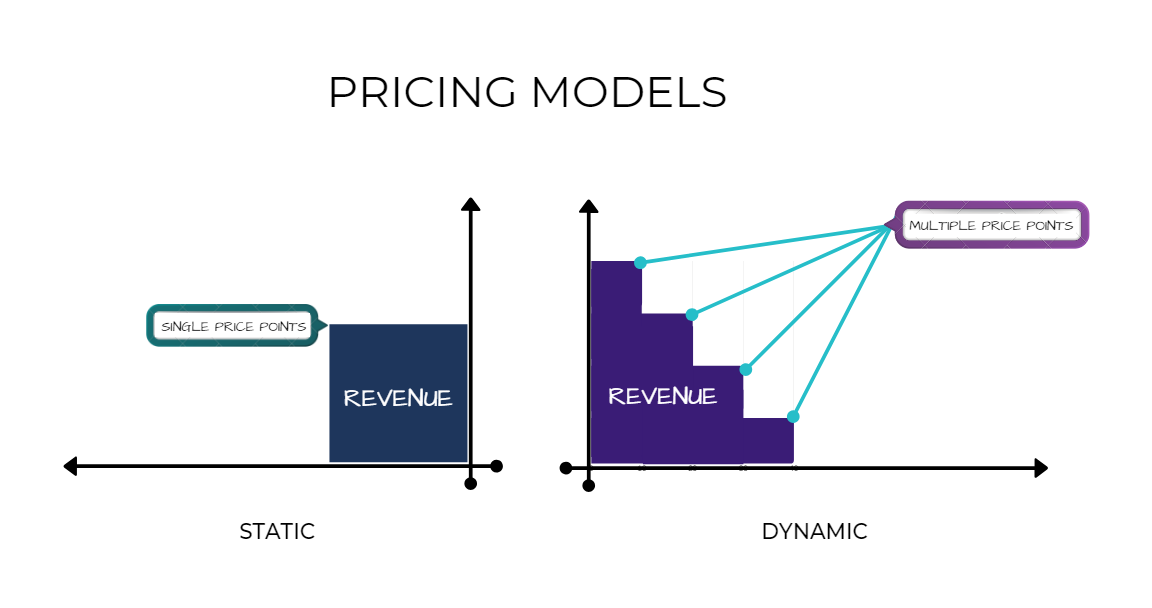
HOW: By collecting data from diverse sources and analyzing it considering customer segmentation and current market environment the solution brings changes to item prices in real-time conditions.
WHY: To ensure business model flexibility and raise the level of customer experience by satisfying actual needs and defining buyer expectations and market tendencies concerning the pricing and provided services.
There are just the most common data science applications in eCommerce and retail but there are much more available and much more to come. The scope techniques ability to bring and analyze running and market insights and perform argumental decision-making constantly and efficiently opened a wide range of possibilities and redefined the term of the quality service.